Data Is The New Dogma: Monetize And Thrive
~6 min read
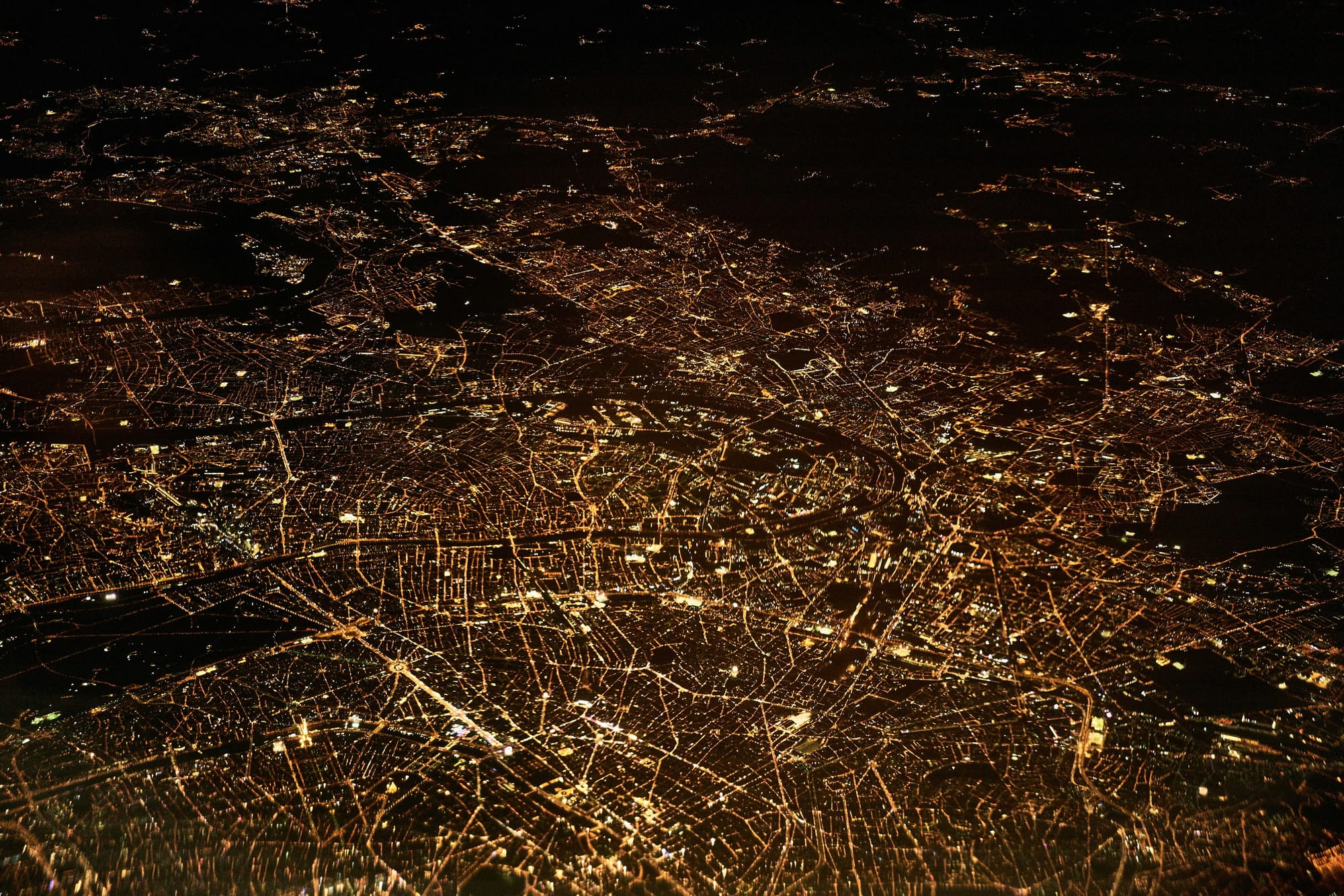
“Data is the new X.” Oil, oxygen, even nuclear power - data’s relevance to the modern economy has led it to be compared to everything under the sun. While it’s true that data is fueling change across virtually every industry, a more apt comparison might capture the fact that data is in fact a byproduct of the larger, ongoing process of digitization, and that once collected, data is not in fact “spent”, but rather stored and transformed. But it’s not enough to merely collect data, and the monetization processes by which businesses turn data into revenue are themselves evolving. As the world continues to digitize, will the data tributaries flow in the direction of revenue for tech giants alone, or are others capable of building sufficiently sophisticated data operations? And as monetization becomes standard practice, which of the various strategies will stand out as worthwhile?
Solving For X
It’s no secret that data can be valuable. But its value must be unlocked via a strategic effort to first target an organizational goal, and then deploy data with the aim of meeting it (or, alternatively, following the steps in reverse by looking for business problems to solve using a particular dataset). But what types of business processes can be readily improved by leveraging data? And moreover, which data monetization strategies are feasible for non-tech firms like telcos or banks who nonetheless have access to plenty of user data?
Earlier this year, researchers at the Technical University of Munich conducted a review of case studies from which they assembled 12 generalized strategies for data monetization. The case studies included monetization efforts by the likes of IBM, Netflix, Walmart, and other notable firms. Not only were common strategies apparent among the case studies under review, but the strategies themselves may be further categorized according to a few basic metastrategies, as well as along more conventional data monetization fault lines like "direct" and "indirect".
Source: Julius Baecker; Martin Engert; Matthias Pfaff; Helmut Krcmar; Mondato Analysis
The strategies uncovered ranged from in-house leveraging of data to create new products or services (or improve existing ones) to outright packaging and selling of data, suggesting that cases do arise in which the best thing to do with your data is to get rid of it. Other strategies fall between the polar extremes of Asset Sale (in which you trade data for cash) and Product/Service Innovation (in which you use data to generate proprietary offerings). Every strategy, however, falls on the continuum between internal and external use. A special consideration for businesses in competitive spaces is how sensible it may be to externalize one’s data when it could be used by a competitor. In such cases, it may be useful to remember limited options, such as: selling some (but not all) of the available data; creating and selling non-reverse-engineerable insights from your data sets; or opening data strategically (via APIs, for example) for mutually beneficial partnerships.
Despite the multitude of ways that data can be put to work, there is always demand for more complete datasets, and firms are also developing new ways to acquire the right data. Amazon, for example, recently launched a “Shopper Panel” program to pay individual users for data on purchases made outside of the Amazon ecosystem. To a data-rich giant like Amazon, individual data is monetizable to the extent that a $10 monthly credit on their platform may be a small price for the data obtained via this novel program. This development comes on the heels of a story published earlier this year alleging that Amazon used data belonging to 3rd party sellers on its platforms for internal product development. Not only would this practice violate Amazon policy -- it would indicate that in the eyes of Amazon, data may be valuable enough to justify risking the company’s reputation for.
The Forest Versus the Trees
In addition to product development, there is one area that big tech firms thrive in when it comes to monetizing their data: advertising. According to the case study review by Baecker et al., the practice of data-driven advertising can be classified under individualization or contextualization.
Individualization is the practice of using data, which is linked to certain customer profiles, “to create value for customers and businesses on an individual basis”. In other words, data about a certain user’s (or account’s) behavior -- whether comprising purchase, browsing, search, or engagement history -- is collected and used to craft a profile of that user. The profile is then used to precisely target the user via individualized recommendations or personalized advertisements, and thereby increase sales.
Contextualization, on the other hand, increases sales by targeting users based on context-related data. Unlike individualization, which relies on individual profiling, contextualization focuses on certain circumstances and targets users based on those circumstances, regardless of individual characteristics. If, for example, a user adds a product to their cart, a contextual targeting service might recommend other products which were often bought alongside by other users.
Data-intensive advertising operations support a massive digital ad industry that represents billions in revenue for firms like Google, Facebook, and Amazon. Based on vast datasets collected from billions of users over years, these firms individually and contextually advertise products on their platforms and earn revenue from impressions, clicks, and sales. The three aforementioned companies comprise an advertising sector that doubled its revenue between Q1 2017 and Q1 2020, and despite COVID’s black swan effects is expected to surpass $70B USD in revenue in Q4 of this year:
Source: Quarterly Earnings, Mondato Analysis
In addition to overall sector growth, a comparative analysis reveals that Amazon’s revenue from advertising (though a small slice of its overall earnings) increased more than six-fold over 14 quarters, eclipsing Facebook’s 2.7x and Google’s 2x respective increases over the same period:
Source: Quarterly Earnings, Mondato Analysis
There are many reasons why Amazon would exhibit faster growth in this area relative to its peers. But nevertheless, the company has found a reliable way to monetize the data collected as a byproduct of its marketplace sales (which, incidentally, account for 4% of all U.S. retail sales). While advertising is not Amazon’s primary profit center, like the company’s cloud business, AWS, it represents a high-growth area that is complementary to the core business. As such, it may serve as a prime example of strategic data monetization at scale.
How The Magic Happens
Clearly, data is of value. Also clearly, businesses ought to at least consider various strategies for monetizing their data. But after determining a path forward to monetization, implementation presents its own set of challenges. First, a data platform able to act as a “single source of truth” (or SSOT) is critical, as data often exists in multiple formats and silos across an organization. Consolidating, standardizing, and creating interfaces for all of an organization’s data on a single platform is the first step toward a robust and holistic monetization operation.
An organization whose data is suitably standardized and centralized will also need an analytics layer to make sense of their data. This will likely include both descriptive and predictive analytic capabilities. Descriptive analytics refers to the ability to understand and summarize historical data, then transform it into a format that is interpretable by humans. Predictive analytics, on the other hand, refers to the ability to estimate likelihood of future outcomes based on probabilities. Together, these capabilities can extract patterns from mountains of data. Then, using prescriptive analytics, these patterns can in turn generate business insights and recommended courses of action.
However, the monetization journey is not as straightforward as it may sound. External considerations like governance, compliance, privacy, and cybersecurity add complexity at every level, with increasing public scrutiny being applied to data operations the world over. And in many cases, analytics capabilities are merely the starting point. The growing field of data visualization deals with the challenge of representing data and insights in accessible, compelling fashion. Even intra-organizationally, data does not stand on its own; business decisions must be argued for, and data-based arguments may fall flat if design and rhetorical considerations are not accounted for (particularly when non-technical personnel are involved in decision-making).
Data may not be the new X after all. Has any resource previously presented businesses with such a breadth of opportunity, while at the same time threatening to stratify the economy along the lines of savvy versus simple? The amount of data at the disposal of big tech, along with their unmatched analytical power, doesn’t exactly spell a level playing field. The good news, however, is that as data becomes more integral to the economy, tools for its monetization (along with other productive uses) are becoming more accessible. Soon, there will be no excuse not to base virtually every decision on some form of data, promising a more efficient use of resources and, ideally, a more prosperous and inclusive world.
Image courtesy of Daniel Bernard
Click here to subscribe and receive a weekly Mondato Insight directly to your inbox.
PropTech: A Forever Home For Fintech
From Ride-Hail To SuperApp, By Way Of Fintech