Synthetic Data Will Transform Fintech AI As We Know It
~9 min read
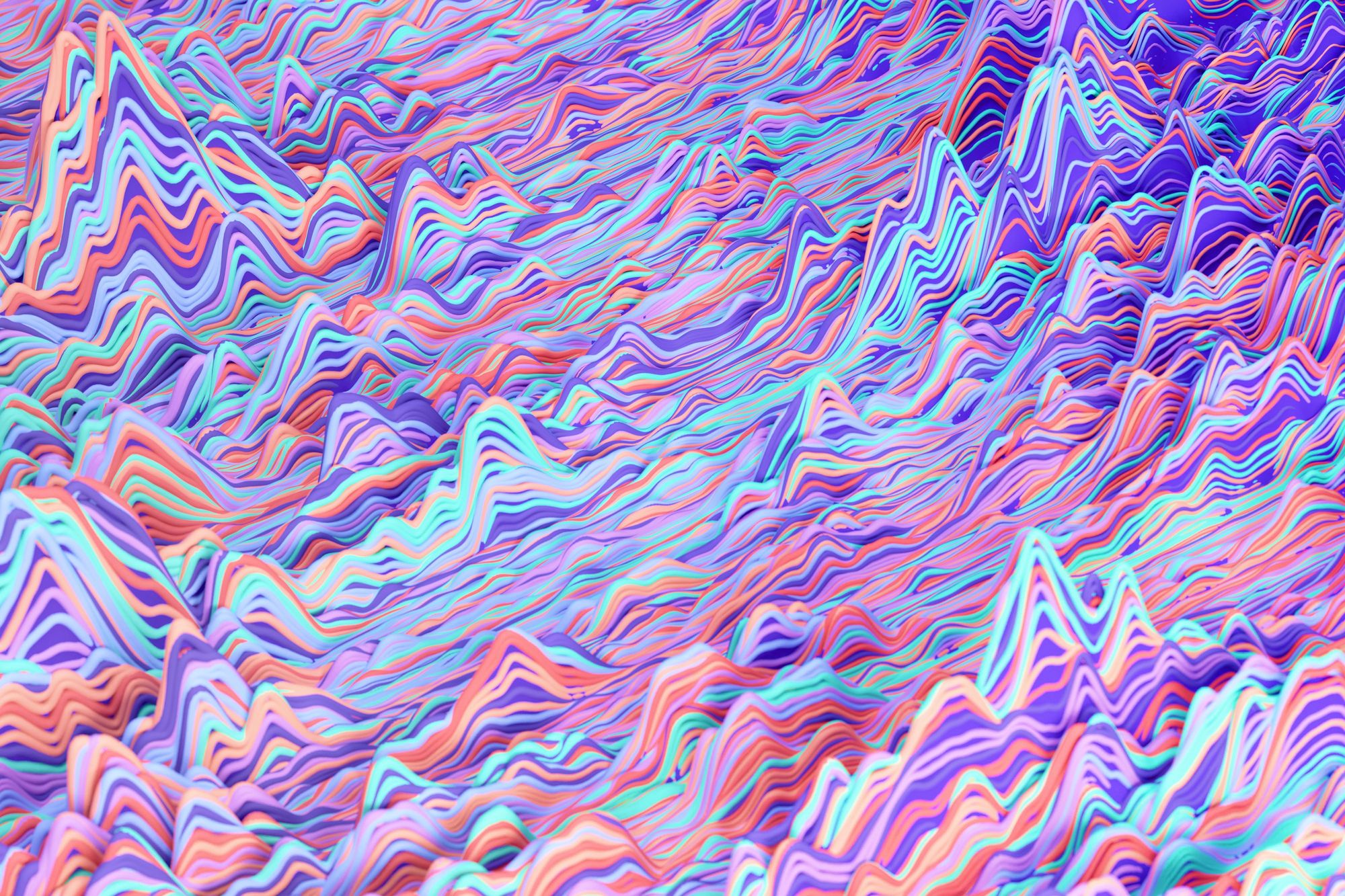
Synthetic data is everywhere, fueling viral innovations splashed across the Internet — yet few have even heard of it. Chat GPT, DALL-E, Stable Diffusion — all of these AI generative tools now capturing the public’s imagination are powered by synthetic data. The advent of synthetic data is happening swiftly and with profound implications for the data economy in areas such as data privacy, sharing and AI fairness. If data is 21st century oil, then imagine a world where financial services companies can create and share accurate data that is not finite, is not privacy-sensitive and adjustable to eliminate historical biases. That world — with both its massive potential in financial services yet unpredictable risks — is what synthetic data will usher in over the next few years.
Realer Than Real
The idea of artificially generated data stretches back decades, but this era of powerful algorithms and artificial intelligence has breathed new life into the concept. Synthetic data in its modern sense differs from mock data: although entirely fake, the kind of synthetic data most pertinent to financial services is derived from real-world data; the other kind of synthetic data is purely generative data that is utilized to feed simulations for training AI programs like autonomous vehicles. Alexandra Ebert is a synthetic data expert and chief trust officer at Mostly AI, a leading synthetic data platform.
“Synthetic data generation technology can be used to automatically learn the patterns and correlations that are present in a data set to then create a completely separate data set. From a statistical point of view, you have nearly the exact same information. You would see similar insights like the same spending behaviors across micro segments. But you don't have any one-to-one relationship between a synthetic individual and your real customers.”
Alexandra Ebert, Chief Trust Officer, Mostly AI
Such innovations allow data to be used by companies, researchers and analysts of these AI algorithms — with its components now properly labeled and explainable to enable human understanding — without violating privacy constraints. Other privacy-enhancing technologies like homomorphic encryption and federated learning are useful for certain purposes, but synthetic data is the most versatile privacy-enhancing tool. It is superior to the various anonymization approaches, which largely employ destructive approaches such as masking, aggregation or randomization. Such tactics do a poor job of mitigating the privacy-utility dilemma: that efforts to protect privacy diminish the utility of a data set, and vice versa.
Not so with synthetic data. As Ebert puts it, synthetic data manages to provide an understanding not of an individual but a generalizable pattern of micro segments of customers. The many barriers that privacy concerns pose to institutions handling regular data no longer apply, and the implications are manifold. Rather than diminishing the data quality for the sake of anonymization — techniques which are prone to deanonymization efforts in our Big Data world — synthetic data is able to power machine learning and AI algorithms in a fashion that requires no compromises on the privacy-utility dilemma.
Source: Mostly AI
Synthetic data fundamentally transforms how organizations view, possess and treat data. Something as straightforward as cloud migration becomes much easier for large financial institutions that otherwise are limited in what data they can migrate. The status quo encouraging companies to hoard data in siloes lacks rationale in a synthetic data-driven world, which offers a GDPR-compliant and replicable data sharing solution.
“We see some organizations that don't only use [synthetic data] on a use case-specific basis and say, ‘we want to train this credit scoring algorithm.’ They think, ‘what are the most valuable assets that we have in our company? Which has the most valuable downstream use cases?’ And they proactively synthesize them internally to make them available for their employees. This is something that definitely sparks innovation.”
Alexandra Ebert, Chief Trust Officer, Mostly AI
Ebert sees synthetic data as potentially unleashing the synergistic potential of data shared both within and across industries to encourage innovation and efficiency. The concern remains that large institutions possessing the most data — and thus the most to lose in a world of democratized, synthetic data — may only double down in hoarding their data, nonetheless. This is where burgeoning open data laws in places like the EU may prove vital in putting an end to such inequalities in data access.
Lukasz Szpruch is professor of mathematics at Edinburgh University and the program director for finance and economics at The Alan Turing Institute, the UK’s National Institute for Data Science and AI, where he researches the responsible adoption of AI in the financial services industry. Szpruch and The Alan Turing Institute are working with the UK’s Financial Conduct Authority to create supplies of synthetic data that agile fintechs — which may have the innovative, tech savvy spirit but lack the hordes of data large financial institutions possess — can use to train their own AI algorithms while remaining privacy-compliant. Szpruch envisions the propagation of synthetic data as setting the stage for standardization of necessary processes shared by all companies.
“There are certain common problems, like detecting bias, combatting fraud, [and] how we make sure that we build algorithms that are fair. It's almost impossible to compare, in a systematic way, what works; there's just too many moving parts. So if we could fix the data, create some common benchmarks that industry would use, that would save billions of dollars and make things so much more efficient.”
Lukasz Szpruch, Director for Finance and Economics, The Alan Turing Institute
While synthetic data’s capabilities in delivering valuable yet privacy-protective data is its greatest strength, its potential use in financial services span several aspects. Of note is its utility in running simulations in training AI algorithms to better react and understand edge use cases, whether that be for detecting fraud or predicting black swan events; synthetic data was employed by governments like the UK at the beginning of the pandemic to better map out, predict and apply solutions to these unprecedented events. Generated synthetic data can fuel machine learning programs with far more data than real data, and at a far cheaper cost.
“What if the payment would have come from here to there? What if the markets would have gone like this? You cannot prepare for unexpected futures by using only real data. Real data is very precious to do the statistical analysis of what happens, but it's not very good to be robust with respect to surprise in the future.”
Manuela Veloso, Head of JPMorgan Chase AI Research
Source: NayaOne
Another important area synthetic data improves upon — if with some caveats — is when it comes to eliminating bias in data sets that are used to train AI algorithms. Because everything in a synthetic data set is easily labelled and explainable — core necessities in employing responsible AI, yet characteristics that self-learning AI algorithms fail miserably at when working with real personal data — it can also be adjusted to account for preexisting biases contained within. Methods like upsampling can properly represent discriminated groups, enriching data without compromising its inherent characteristics.
From Too Little To Too Much?
Even with its potential benefits in so many core issues affecting AI training and development, synthetic data is not a silver bullet altogether. Starting with the case of eliminating bias and promoting fair AI algorithms, experts like Ebert and Szpruch view synthetic data as an important tool, but it does not solve these issues altogether; what fairness truly entails is a question that goes beyond the capabilities of programmers and self-learning AI algorithms to require the perspective of lawyers and even philosophers. The tweaking of data sets to create a more just, representative world to run AI programs on is easier said than done.
Such delicate processes take place in a context where the barrier to entry in producing synthetic data is low, but differences in quality can be significant.
“It's very easy to produce synthetic data; it's very hard to produce synthetic data that has required properties for the specific use case that you care about and that gives you guarantees around its privacy.”
Lukasz Szpruch, Director for Finance and Economics, The Alan Turing Institute
Synthetic data has seen a particular explosion in the past two years, with now approximately 100 companies offering synthetic data products and services as more companies seek privacy-compliant data to fuel their AI programs. Yet at the moment, Szpruzch says it can be hard for clients to know just how good individual companies and their synthetic data generators really are. According to Szpruch, it’s impossible to be 100% privacy-protecting while retaining 100% of the derivable insights from the original data; it still requires a delicate balance in design and implementation of these solutions that aren’t necessarily properly vetted in these early stages of synthetic data development.
These traits highlight the nature of synthetic data as a tool that can be subject to nefarious manipulation or outright poor design. Synthetic data’s transcendent ability to democratize data and make a once-scarce resource so numerous will also come with the downsides digital society is all-too familiar with by now; another prominent example of synthetic data? Deep fakes.
“We will soon be in the situation where there will be data sets everywhere. The challenge will be to be able to tell which are fake and which are real… The main challenge would be how to make sure that you can trust these data sets and that this data is representative. This is not a replacement of the (original) data — it needs to be used in combination with it.”
Lukasz Szpruch, Director for Finance and Economics, The Alan Turing Institute
Utopian Dystopia
The profound excitement yet underlying concerns surrounding synthetic data is following a textbook process: democratization through digital means, whether that be the information superhighway itself or expression on social media, saw initial, utopian origins eventually swamped by misinformation and distortion at the hands of malevolent actors. Through that lens, the propagation of synthetic data will likely be a mix of these extremes as well — only applied to the various kinds of data undergirding digital society.
These transformations are coming sooner than you think. Spurred on by the simultaneous need for granular data while remaining compliant with data privacy laws, synthetic data is booming. Gartner went so far as to predict that by 2024, 60% of data used to develop AI will be synthetically generated — an estimate that some experts find to be a bit overblown, but which captures just how critical synthetic data will be moving forward.
At this juncture, synthetic data has seen the most adoption among traditional financial institutions who must develop AI-driven solutions while remaining compliant with stringent data privacy regulations. According to Ebert, eight of the top 10 banks in the U.S. are already using synthetic data, either for experimentation or to apply towards their AI algorithms powering areas like lending, analytics, fraud detection, marketing and product development and testing.
Younger fintechs have been slower to adopt synthetic data, and the reasons are twofold. Such startups lack the real data — or the yet-to-come democratized data regimes to level the playing field — necessary to synthesize data for attaining insights and training AIs. Even among those that do, they don’t possess the same privacy concerns and requirements that incumbents grapple with. However, especially as fintechs mature and progress to later funding rounds, the pressure from investors to have tight privacy and data standards are compelling them to explore synthetic data solutions. Pressure on smaller institutions will intensify as regulations such as the EU’s AI Act are implemented, all but requiring institutions to employ synthetic data if they wish to sufficiently feed AI algorithms with granular yet sensitive data while adhering to privacy and bias standards.
Beyond fintechs in developed markets, synthetic data will certainly spread to financial services in emerging market contexts as well. Even if AI regulations are slow to arrive, the superior cost of data acquisition through synthetic means will attract fintechs at the vanguard of artificial intelligence. The ability to augment and enrich insufficient real data sets synthetically can expand access and inclusion without relying on alternative data sources that violate the privacy of thin-file customers. Adjusting exported data sets to fit local conditions can conceivably ease expansion into new markets easier for fintechs — though this will require careful design and validation to ensure data remains fitted for local contexts.
Ultimately, no corner of artificial intelligence in the digital financial services space will be left untouched by synthetic data. The superior solution synthetic data provides for so many of AI’s current shortcomings — and the potential for democratization it contains — in a cost-effective manner renders its ascension in financial services a given. To be sure, synthetic data remains a young area requiring further research and development. Tremendous risks lie ahead — it will be improperly used, created and applied, and in unpredictable ways — but it will help reorient data regimes as we know them, with trickledown effects throughout financial services and beyond. In a very real sense, transformation is coming.
Image courtesy of Maxim Berg
Click here to subscribe and receive a weekly Mondato Insight directly to your inbox.
Are Colleges Failing At Blockchain?
What’s Missing in Affordable Housing Financing